Fraud-busting in the new ‘normal’: keeping costs and false positives down post-COVID
Fraudsters are profiting from the pandemic, while financial firms’ fraud-detection systems are swamped with false positives. As firms adjust to a new ‘normal’, graph analytics and supervised and unsupervised models can help them keep pace with criminal behavior.
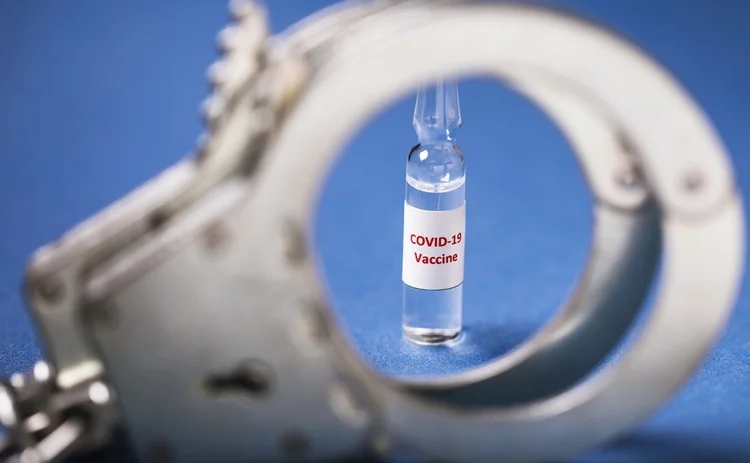
The evolving fraud landscape
In modeling and mitigating the risks of the coronavirus pandemic, some machine learning (ML) strategies have had notable successes, in areas such as algorithmic execution and fund management. ML fraud-detection systems, however, have coped less well amid the turbulence and mounting panic. ML anti-fraud models often use anomaly detection, and are constructed on a baseline of ‘normal activity’ against which anomalies and unusual behavior can be detected.
But in the new era of COVID-19, spending patterns have changed markedly. With every major economy in freefall and billions of people under movement restrictions, the crisis is driving global growth in e-commerce, as millions of consumers purchase goods, services and entertainment online. Compared with the same time last year, transaction volumes in most retail sectors increased by 74% in March. The crisis has highlighted the need for faster real-time access to digital payment services for individuals and businesses in every jurisdiction.
The growing ubiquity of digital payments means that fewer transactions are cash-based – and as cashless transactions go up, so do attempts at fraud. The UK’s National Cyber Security Centre (NCSC) reported that it has so far detected 2,500 online COVID-19 scams, including fake online stores selling virus-related items. According to the latest figures from UK fraud-reporting center Action Fraud, at least 824 people in the UK have fallen victim to COVID-19 related scams, with total losses of nearly £2m so far this year. Fraud is also a persistent threat to financial institutions (FIs), and banks have been reporting a significant rise in both internal and external fraud during the crisis.
Regulatory pressure
In March, the European Banking Authority (EBA) issued a policy statement reminding banks to ‘maintain effective systems and controls to ensure that the EU’s financial system is not abused for money laundering or terrorist financing purposes’ during the pandemic. It also called on regulators to support FIs’ ongoing anti-money laundering (AML)/combating the financing of terrorism (CFT) efforts, and reminds readers that financial crime remains unacceptable, even in times of crisis.
Many regulators, including the FCA, have encouraged banks to leverage innovative technology, such as ML-based techniques, in the fight against financial crime. While FIs are encouraged to experiment with artificial intelligence (AI) and ML, however, they must still be able to adequately explain and validate new risk-assessment models to regulators. Likewise, the FCA has warned that FIs will be fined if they adopt vendor-supplied systems that are not adequately tailored to match the size and complexity of their business. So while regulators are encouraging innovation, they need to be comfortable that ML systems are effective.
False starts
In many FIs, analytical systems for anti-fraud models are ill-prepared for the sharp rise in digital transactions we are seeing, because the data they rely on is becoming outdated and obsolete. Fraud-detection strategies, which rely on past patterns of behavior to make predictions, are struggling to cope as the concept of ‘normal’ is completely upended. As ‘unusual account activity’ appears to increase, fraud-detection systems are producing dramatically more false positives.
ML-based fraud detection is a complex process (see Figure 1), in which analysts interpret patterns in data and apply data science to continually improve a system’s ability to distinguish normal from abnormal behavior. Large datasets must be analyzed to glean information about the latest threats, anomalous activities and potential security incidents, and thousands of computations must be performed in milliseconds. Data overload, which occurs when the level of input into a system exceeds its processing capacity, can create an incomplete view of risk exposures, obscuring the patterns and behaviors required to develop predictive capabilities.
Figure 1. A complex process: an anti-fraud system and its anomaly-detection capabilities
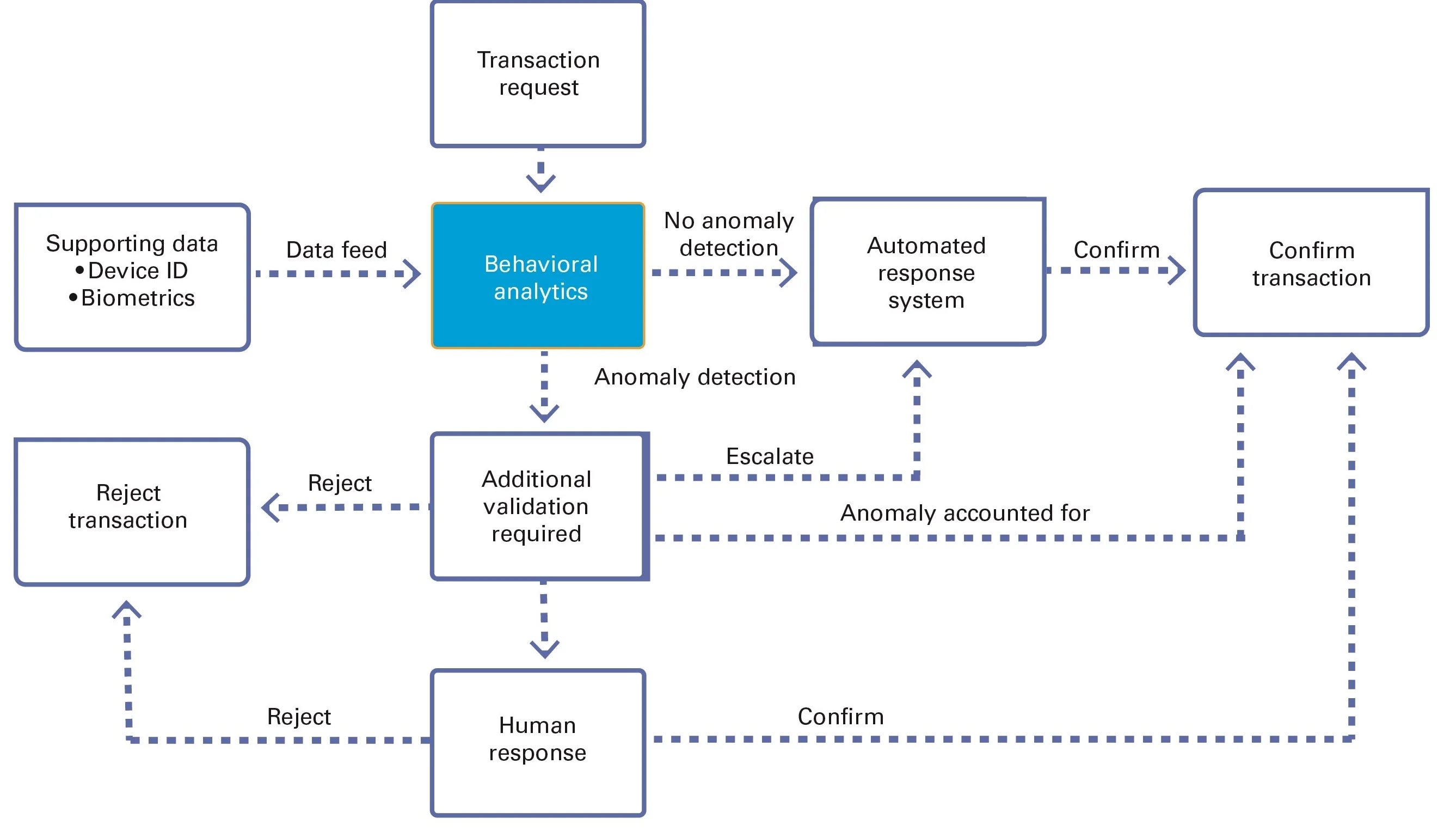
Source: Chartis Research
The new wave of post-coronavirus false positives has created an opportunity for fraudsters to carry out their activities with little chance of detection. And the combination of a rise in fraudulent activity and more false positives has added hugely to fraud-prevention executives’ workload – creating the need for more, and more costly, manual investigations. But while there are now more alerts and more false positives, reduced headcount in many FIs means that fewer investigations are taking place, and at a slower rate than before. Behavioral monitoring systems, therefore, must be more reactive to capture fraud actors. Otherwise more results will have to be delivered to the human response team, a process that is both costly and difficult to resource in an environment that requires social distancing.
Redefining fraud models in the new ‘normal’
Looking ahead, FIs face a key challenge in adapting their fraud-detection systems so they can establish effectively what ‘normal’ activity or behavior is at any given point in the future. As lockdown restrictions ease in several countries over the coming months, the concept of ‘normal’ is likely to alter significantly. And as the number of digital transactions continues to grow rapidly, so too will the need for FIs to retrain their systems and reconfigure groups of individuals and their spending activity faster and more often than before.
‘Supervised’ models used by most FIs detect fraud using directly relevant, tagged transaction data. ‘Unsupervised’ models employ a form of ‘self learning’ to detect behavioral anomalies, by identifying transactions that differ in some way from most of the others being processed. By combining supervised and unsupervised models, FIs could detect fraud more accurately: while supervised learning is predictive, unsupervised learning can distinguish anomalous behavior in instances where tagged transaction data is relatively sparse or non-existent. In essence, the algorithms look for new events that follow unprecedented patterns, such as the rise in digital transactions during the COVID-19 pandemic.
To further sharpen the distinction between a false-positive event and a false-negative one, and to assess risks and behaviors in real time, FIs should leverage advanced analytics. Predictive analytics help to make systems more sensitive to evolving fraud patterns by automatically adapting to recently confirmed fraud cases – providing a more accurate separation between cases of fraud and non-fraud.
Graph analytics, also known as network analysis, can be a powerful ally in improving the performance of fraud-detection systems. These tools examine how entities connect and relate, and provide insights into how networks operate as a whole. To detect fraud accurately, systems must instantaneously understand connections and identify anomalies in the links between entities, transactions, payment methods, locations, devices and transaction times. Graph networks contain nodes, representing modeled entities, and these are connected by edges, which capture the relationships between entities (see Figure 2). By treating entity relationships as graphical representations, graph analytics tools can assess their various elements, such as the distance between two individuals or places. FIs can use more complex graphs, involving millions of nodes or trillions of edges, to analyze large and complex datasets of entities with multiple relationships.
Figure 2: Example graph analytics model, highlighting links and relationships between entities
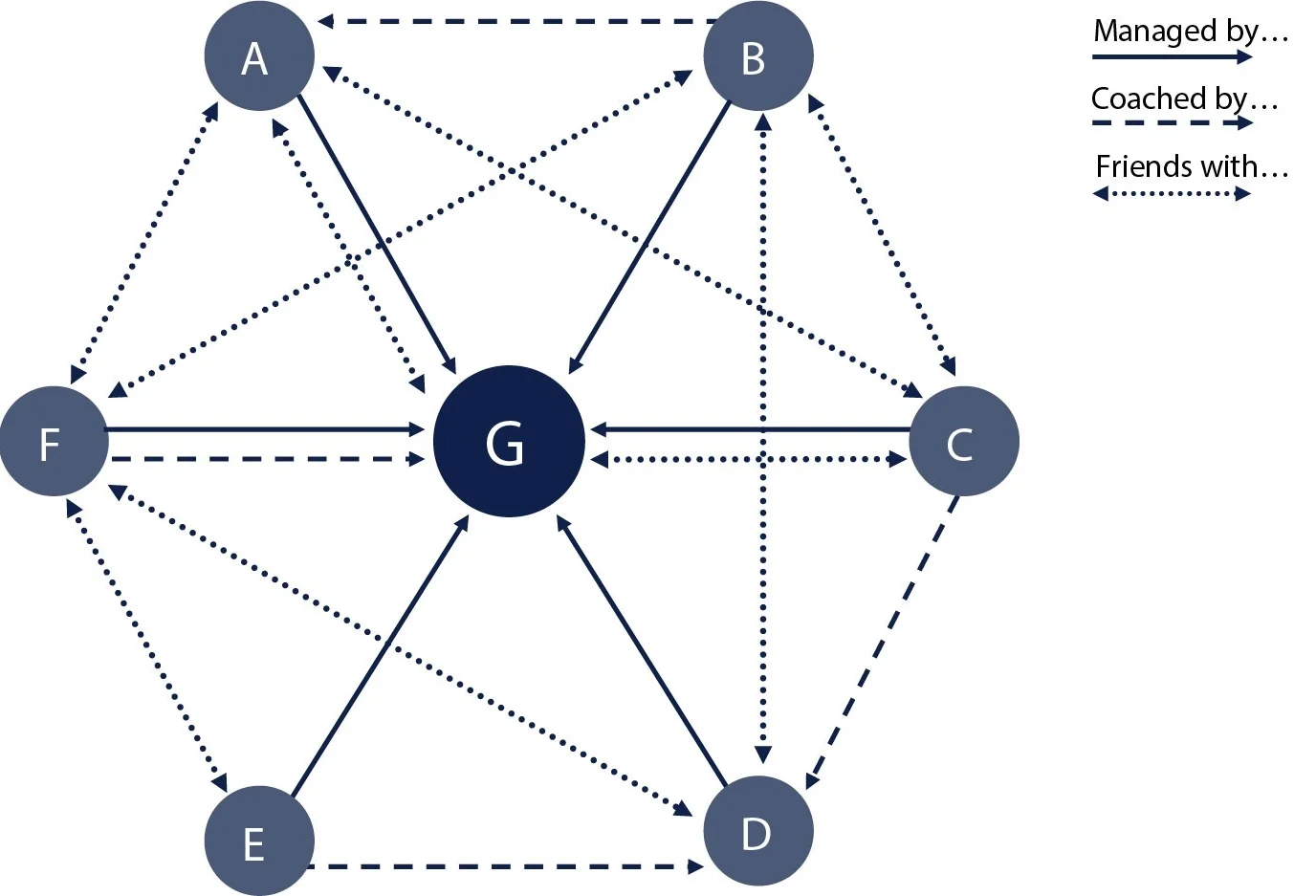
Source: Chartis Research
A multi-layered and flexible approach
The far-reaching consequences of the COVID-19 crisis are uncertain, but FIs’ ability to quickly identify new threats and adapt their fraud-prevention controls will be critical to ensure continuity of business operations. The pandemic has highlighted the need for flexible technology architectures, and the effectiveness of FIs’ efforts to mitigate their risk exposure will depend ultimately on their willingness to develop new technology strategies and invest in systems and maintenance.
As criminals become more sophisticated, a multi-layered approach for fraud-detection models is crucial to help reduce false positives and save costs. If FIs can create more reactive behavioral-monitoring systems, and invest in advanced analytics and a combination of supervised and unsupervised models, they have a good chance of mitigating their risk exposure and reducing the amount of money they spend on manual investigations. In addition, as the nature of business changes rapidly, FIs will have to perform AML and Know Your Customer (KYC) checks much more quickly and effectively. In this context, due-diligence measures cannot be compromised, and reliable, trusted data and flexible workflow tools are paramount in managing the onboarding process.
Further reading
- The Technology Impacts of COVID-19 - Market Overview and Guidance
(2020, Chartis)
- Enterprise Fraud: Market Update and Vendor Landscape, 2019
(2019, Chartis)
- Beyond Detection: Driving Automation and Artificial Intelligence info Financial Crime Risk Management
(2019, Chartis)
- Spotlight on Graph Analytics in Finance
(2018, Chartis)
Points of View are short articles in which members of the Chartis team express their opinions on relevant topics in the risk technology marketplace. Chartis is a trading name of Infopro Digital Services Limited, whose branded publications consist of the opinions of its research analysts and should not be construed as advice.
If you have any comments or queries on Chartis Points of View, you can email the individual author, or email Chartis at info@chartis-research.com.
Only users who have a paid subscription or are part of a corporate subscription are able to print or copy content.
To access these options, along with all other subscription benefits, please contact info@risk.net or view our subscription options here: http://subscriptions.risk.net/subscribe
You are currently unable to print this content. Please contact info@chartis-research.com to find out more.
You are currently unable to copy this content. Please contact info@chartis-research.com to find out more.
Copyright Infopro Digital Limited. All rights reserved.
As outlined in our terms and conditions, https://www.infopro-digital.com/terms-and-conditions/subscriptions/ (point 2.4), printing is limited to a single copy.
If you would like to purchase additional rights please email info@chartis-research.com
Copyright Infopro Digital Limited. All rights reserved.
You may share this content using our article tools. As outlined in our terms and conditions, https://www.infopro-digital.com/terms-and-conditions/subscriptions/ (clause 2.4), an Authorised User may only make one copy of the materials for their own personal use. You must also comply with the restrictions in clause 2.5.
If you would like to purchase additional rights please email info@chartis-research.com