Statistical tools: a new way to beat white-collar fraudsters
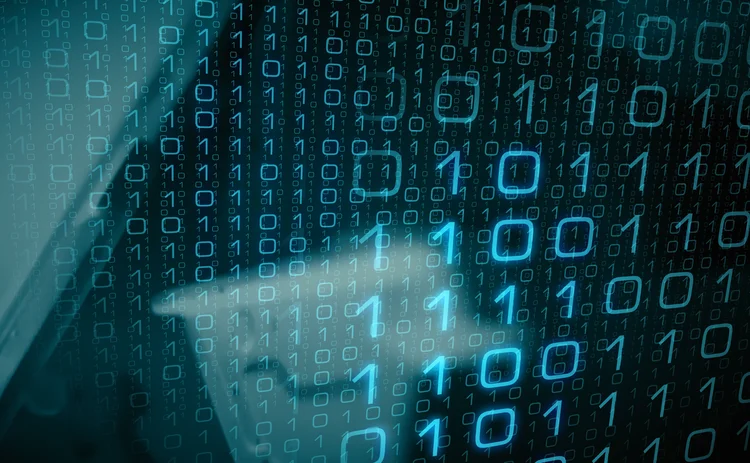
The stubborn persistence of accounting fraud
Business accounting fraud has been a serious issue for some time, with notable high-profile examples from the relatively recent past including Enron Corporation (2001) and WorldCom (2002). But despite the repercussions of these and other events, and the strong regulatory response in the form of the Sarbanes-Oxley Act (2002), accounting fraud has not disappeared. Instead, it has adapted, becoming more sophisticated and elusive. Recent cases highlighted by independent researchers (such as those involving the Tingo Group, the Adani Group and Welltower), show that bad actors continue to find new ways to manipulate financial data, even in the face of enhanced regulatory scrutiny.
Better tools: the Beneish model
Faced with the clear challenge posed by accounting fraud, firms are constantly on the lookout for new tools they can use to mitigate the problem. The Beneish model, conceived in 1999 by Professor M. Daniel Beneish at Indiana University, was the first tool to address accounting fraud and has become an increasingly vital methodology for detecting earnings manipulation.
Leveraging financial ratios and eight key variables (see Table 1), the model provides a systematic approach to identifying fraudulent activities. Collectively, its variables form an ‘M-Score,’ a quantitative measure of the likelihood that a company has manipulated its earnings. By closely analyzing the variables, analysts can identify potential accounting fraud more effectively.
Table 1: The variables employed in the Beneish model, and how each can be used in identifying manipulative accounting practices
Variable | Description | Role |
Days’ Sales in Receivables Index (DSRI) | Measures the change in days’ sales in receivables. | High DSRI may indicate aggressive revenue recognition, a red flag for fraud. |
Gross Margin Index (GMI) | Measures the change in gross margin percentage. | Deteriorating GMI may suggest the potential manipulation of profit margins. |
Asset Quality Index (AQI) | Measures the change in asset quality. | A declining AQI may signify the use of lower-quality assets to inflate earnings. |
Sales Growth Index (SGI) | Measures the change in sales growth. | A high SGI in the absence of increased cash flow may point to revenue manipulation. |
Depreciation Index (DEPI) | Measures the change in depreciation expense. | A rising DEPI may indicate an effort to reduce depreciation to boost profits. |
Selling, General and Administrative Expenses Index (SGAI) | Measures the change in SG&A expenses. | A sharp increase in the SGAI may signal that costs are being understated to inflate profits. |
Leverage Index (LVGI) | Measures the change in leverage. | An escalating LVGI may suggest an increased reliance on debt to hide financial troubles. |
Total Accruals to Total Assets (TATA) | Measures total accruals relative to total assets. | A high TATA ratio can indicate an increase in non-cash adjustments, raising the suspicion of earnings manipulation. |
Source: Chartis Research
Limitations of the Beneish model
While the Beneish model offers a valuable tool for fraud detection, its limitations warrant consideration. A 2015 study by Herawati highlighted that certain variables within the model, such as the sales index, asset quality index and leverage index, did not demonstrate consistent significance in identifying fraudulent activity across all industries. This underscores the need for further research and development to refine the model and adapt it to the specific characteristics of different sectors. Herawati also advocates for the integration of data mining techniques alongside the Beneish model, to enhance the accuracy of detection by uncovering hidden patterns and anomalies that are not readily apparent via traditional ratio analysis. By acknowledging and addressing these limitations, the field of statistical fraud detection can continue to evolve and refine its strategies for safeguarding the integrity of financial markets.
Emerging machine learning techniques
Recent advances in machine learning (ML) are pushing the boundaries of fraud detection in financial statements. A 2023 study by Fan et al sheds light on promising new approaches that are using predictive models, and which go beyond the analysis of financial data. Fan suggests that sentiment analysis could be used in news articles surrounding a particular company to augment traditional ratio-based models. By detecting shifts in news sentiment around key financial terms, or by highlighting negative press coverage related to accounting practices, ML algorithms could flag suspicious companies even before warnings appear in financial statements. This opens up exciting possibilities for proactive fraud detection that could help investors, auditors and regulators stay ahead of increasingly sophisticated fraud. But further research is necessary to refine these models and assess their real-world efficacy, to ensure the fair and accurate identification of potential fraud without false alarms.
Fortifying financial markets: the role of accounting fraud detection solutions
The industry’s recognition of the Beneish model’s importance is leading to a collective effort to incorporate it into novel fraud detection products. This move will not only safeguard the interests of clients but also reinforce the credibility and trustworthiness of listed company investments.
Accounting fraud detection tools can help to strengthen the following roles, tasks and workflows:
- Audit. Internal and external auditors can use accounting fraud detection solutions as an early warning system to catch fraud threats early. Solutions can screen financial statements for fraud as an initial check or can be used as validation tools to ensure that nothing has been missed by a post-audit. Given their central role in the financial reporting cycle, auditors are emerging as the earliest adopters of these tools.
- Risk professionals. Risk professionals in due diligence and counterparty risk can leverage accounting fraud signals to detect and remediate any risk exposures their firm may face. This is an emerging (but so far not widely used) use case that is likely to be less applicable to the financial services space (for counterparty risk, for example). It could be helpful for due diligence in bank lending, however, or other points of intersection with corporate and small and medium-sized enterprises (to support commercial and corporate or transaction banking).
- Investors. Thematic funds and short-selling hedge funds are the most likely users of accounting fraud detection tools. Indices and portfolios can be constructed on the quantitative side using accounting fraud scores. On the fundamental side, as in auditing, accounting fraud signals can be used to tip off investigative short sellers about possible risks.
The solution capabilities of currently available accounting fraud tools include:
- Accounting risk typologies and control assessments. These solutions help users understand a range of accounting fraud typologies and how to detect them.
- Statistical risk scoring. These tools enable users to identify indicators of accounting fraud derived from typologies.
- Fraud risk watchlist monitoring. These tools allow users to monitor a list of companies and flag risks when new financial disclosures are available. Pre-packaged datasets are available to enable firms to monitor listed companies. But users should also be able to upload their own documents for portfolio companies or clients to have them scored.
By advocating for the widespread adoption of these sophisticated solutions, fraud departments can play a pivotal role in securing the financial interests of clients and elevating the industry’s ethical standards. The development and implementation of tools must be not only profit-driven but also ethical, responsible and secure.
Looking ahead: fraud accounting and ESG
Chartis believes that industry experts should consider integrating accounting fraud detection within environmental, social and governance (ESG) frameworks. ESG considerations have gained prominence in investment decisions, and the governance component encompasses fraud risk. By incorporating such fraud detection tools as the Beneish model into ESG assessments, firms could significantly enhance the precision of their financial risk evaluations.
Furthermore, the emerging role of ESG technology as a potential avenue for short-selling strategies warrants exploration. Industry experts can actively investigate how ESG data sources, including those pertaining to fraud, can be harnessed for more informed and responsible investment decisions.
Only users who have a paid subscription or are part of a corporate subscription are able to print or copy content.
To access these options, along with all other subscription benefits, please contact info@risk.net or view our subscription options here: http://subscriptions.risk.net/subscribe
You are currently unable to print this content. Please contact info@chartis-research.com to find out more.
You are currently unable to copy this content. Please contact info@chartis-research.com to find out more.
Copyright Infopro Digital Limited. All rights reserved.
As outlined in our terms and conditions, https://www.infopro-digital.com/terms-and-conditions/subscriptions/ (point 2.4), printing is limited to a single copy.
If you would like to purchase additional rights please email info@chartis-research.com
Copyright Infopro Digital Limited. All rights reserved.
You may share this content using our article tools. As outlined in our terms and conditions, https://www.infopro-digital.com/terms-and-conditions/subscriptions/ (clause 2.4), an Authorised User may only make one copy of the materials for their own personal use. You must also comply with the restrictions in clause 2.5.
If you would like to purchase additional rights please email info@chartis-research.com