No surprises: 2025 will be another big year for AI
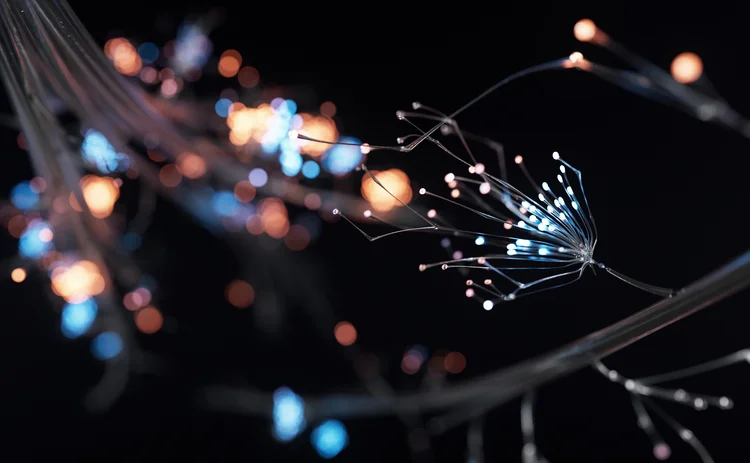
It’s no great secret that 2024 was a huge year for AI. Major research labs released new models that captured headlines and the attention of industry. OpenAI’s o1 model, for example, designed to tackle reasoning tasks, is now stimulating discussion about the impact that ‘chain of thought’ reasoning can have on model performance. Meanwhile, multimodal capabilities continue to develop: Meta has expanded its open-source Llama model lineup, while Google has unveiled more of its Gemini series. And for many developers, Anthropic’s Claude 3.5 Sonnet has quickly become the coding model of choice.
Beyond Llama, the open-source community also had its share of noteworthy releases last year, with models such as DeepSeek-V2 and Qwen2.5. Both were developed in China, where access to the latest AI chips is restricted, reinforcing the adage that necessity is the mother of invention. These contributions, alongside techniques such as distributed learning, continue to fuel the open-source AI ecosystem, even as market leaders such as OpenAI continue to adopt a firmly closed-source approach.
The open- versus closed-source debate remains particularly fraught when it comes to AI safety. On one side, advocates argue that transparency fosters innovation and oversight. On the other, critics warn that open-sourcing AI models could hand potentially dangerous tools to bad actors, both state-backed and individual.
Alongside the debates about technical and governance issues, the financial stakes in the AI race have skyrocketed. Big Tech’s capital expenditure on AI has soared, and significant VC dollars continue to be funnelled into the sector. And as in other industries, vendors and institutions in financial services are eager to demonstrate that their research and development efforts are keeping pace with cutting-edge advances. (Though it is worth noting that, in the past, the sector has been awash with waves of AI optimism.) Moreover, the rush to build new data centers – and secure the energy sources to power them – has also escalated.
My personal optimism for the fate of underdogs in the sector was given a boost by the windfall enjoyed by the island of Anguilla in 2023, following huge sales of the .ai domain. Yet even with a few surprises, one constant remains: scale is the key differentiator when it comes to AI. In a reminder of Rich Sutton’s Bitter Lesson, companies that can source large datasets and harness the largest amount of computing resources are likely to gain a competitive advantage and huge market share.
Predictions galore
Against this background, 2025 has already ushered in plenty of predictions for the AI industry. These range from forecasts about which AI trends will have the biggest impact on industry to debates about how close we are to achieving artificial general intelligence (AGI), as well as how all this will revolutionize the workforce.
Of course, this propensity for prediction isn’t limited to AI – the year began with the usual market and economic forecasts. The tug of war between interest rates and inflation continues, and for most commentators and participants, guessing how markets will respond is top of the agenda. And given the importance of the ‘Magnificent Seven’ – the dominant US technology companies that are driving the performance of the S&P index – market predictions and AI forecasts have become increasingly intertwined.
No doubt this latest period of evolution will provide important and valuable lessons in misplaced market optimism and tech boom-and-bust cycles. In retrospect, we might analyze this period with the same fascination we devote to picking apart the spread of the internet, the rise of personal computing and the expansion and implosion of the dot-com bubble.
What to consider in 2025 (for now…)
In lieu of more pithy projections, here are a few things to consider as generative AI (GenAI) projects and applications mature:
- Stay focused on the usefulness of applications. For many AI research labs, building AGI remains the current big goal. But for most firms involved in AI solution development, including software as a service (SaaS) projects, practical and valuable AI applications should remain the primary focus. New product releases and benchmarking results may be exciting, but successful projects will hinge on how well they deliver what’s useful and feasible. Currently, for example, amid a deluge of SaaS copilots, coding assistants are by far the most popular application of GenAI by financial institutions. And as firms grapple with the transition from proof of concept to production, the most promising projects are those that address extensive data retrieval and document parsing in specific use cases, such as loan processing or regulatory intelligence.
- Pricing GenAI applications is difficult. There is little consensus around how to price GenAI services effectively. Even the big AI labs are struggling to balance consumers’ access to their models with long-term business sustainability. In the risk management space, AI models have long been a feature of many products, but companies often launch new GenAI initiatives expecting to generate dedicated revenue streams. While some projects will succeed, others may force businesses and customers to ask: ‘was the investment worth the reward?’
- Retrieval-Augmented Generation (RAG) techniques are evolving. Although RAG has become something of a buzzword, under the current AI paradigm it remains the clearest way to reliably reference internal and novel data in GenAI projects. Ongoing advances in RAG technology, as well as best practices that are starting to emerge (such as using specific embedded models and vector/knowledge graphs) are solidifying its role as an essential tool for enterprise AI applications.
- AI innovations are not limited to the latest and largest foundation models. Innovations in model architecture and training techniques can have broad impacts and are often adopted quickly by industry practitioners. Take ModernBERT, for example, an updated version of the BERT language model released in December. Despite being a mainstay in the industry, in recent times the original BERT has been neglected. However, the new model addresses issues around task efficiency and longer text sequence windows, improving both performance and speed. Alongside state of the art technology, developers also benefit from a growing ecosystem of tools and frameworks to support their application development. These include serverless application programming interfaces (APIs) with optimizations (such as autoscaling).
For more AI insights and analysis, see our most recent AI report, available here.
Only users who have a paid subscription or are part of a corporate subscription are able to print or copy content.
To access these options, along with all other subscription benefits, please contact info@risk.net or view our subscription options here: http://subscriptions.risk.net/subscribe
You are currently unable to print this content. Please contact info@chartis-research.com to find out more.
You are currently unable to copy this content. Please contact info@chartis-research.com to find out more.
Copyright Infopro Digital Limited. All rights reserved.
As outlined in our terms and conditions, https://www.infopro-digital.com/terms-and-conditions/subscriptions/ (point 2.4), printing is limited to a single copy.
If you would like to purchase additional rights please email info@chartis-research.com
Copyright Infopro Digital Limited. All rights reserved.
You may share this content using our article tools. As outlined in our terms and conditions, https://www.infopro-digital.com/terms-and-conditions/subscriptions/ (clause 2.4), an Authorised User may only make one copy of the materials for their own personal use. You must also comply with the restrictions in clause 2.5.
If you would like to purchase additional rights please email info@chartis-research.com